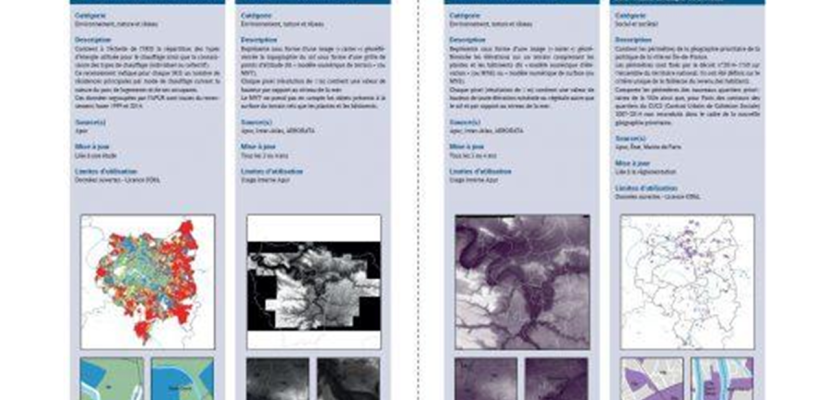
Conversational intelligence to support the production of high-quality open data

About this good practice
A major challenge for data hosting platforms is the quality of data uploaded from several co-producer sources.
They may be in different formats, described using different thought patterns or words, which may prevent the user from identifying their interest at first glance.
Data catalogues are tools that can be made available to help:
providers publishing usable data
players identify data of interest
Data providers have to provide metadata to describe the content of the dataset. This includes a description of the geographical scope, the last update and frequency, a description of the uses made and feedback. On the DataGrandEst platform, these catalogues have been set up for geographic data. But some are incomplete or inconsistent with the actual content..
As part of the regional strategy for open public data, DataGrandEst (policy instrument) co-developed a digital tool to further improve the quality of the catalogues. It is able to provide automated feedback to data providers to help them publish high quality and usable data.
The tool is based on scripts to query geographic data catalogues using their APIs (Application Programming Interface). It reduces the cost and facilitates the downstream development of services. A new version is currently being studied using:
AI solutions to extend the lexical analysis and to link up user with similar interests
NLP solutions, to enhance the description of datasets in terms of what they contained and what could be extracted from them.
Resources needed
Human resources from DataGrandEst with the support of a service provider to automate the process (6 man months equivalent to €30k)
Evidence of success
The tool delivered consistent and accurate data:
- +80% quality
- less redundancy of OD
- greater visibility to choose the right dataset to use
This facilitates the downstream development of services and helps to reduce data cleaning cost for users. Various studies estimate a 10-fold reduction (different for each data user).
By encouraging users to comment on their uses and developments, the tool has enabled the creation of a community and helped to drive it forward (users' number increased).
Potential for learning or transfer
Relevance: Data quality automation concerns any data hosting platforms
Replicability: The tools are easily replicable. Check beforehand that the solution is compatible with the platform's catalogues and datasets.
Warning: technical skills are needed to implement the tools and check performance
Sustainability: The tools run once a day in just a few seconds = low resource consumption.
DataGrandEst evaluated a real interest to develop further the solution, looking at what could be achieved with high-performance proprietary solutions, if they exist? We would need to develop the scripts to make all the building blocks work together. We also need to be able to add verification rules. AI solutions could be interesting, for example, for filling in catalogue fields.
We have also enabled users to discuss how they use the data and how they can improve it. AI could be used to link up users who could work together based on those descriptions.